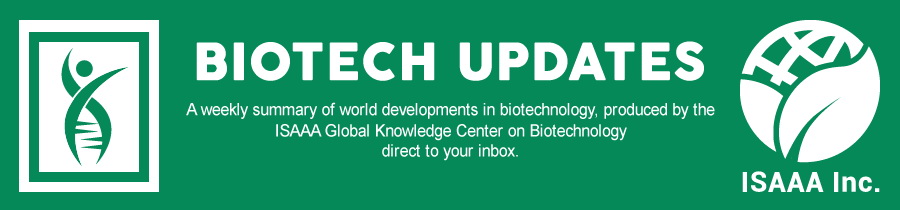
Machine Learning Model Could Help Reduce Agricultural Greenhouse Gas Emissions
May 11, 2022 |
A team led by researchers from the University of Minnesota's Digital Agriculture Group has significantly improved the performance of numerical predictions for agricultural nitrous oxide emissions. The researchers developed the first-of-its-kind knowledge-guided machine learning model for agroecosystem called KGML-ag.
KGML-ag was constructed using a special procedure that incorporates knowledge from an advanced agroecosystem computational model, called ecosys. It includes less obvious variables such as soil water content, oxygen level, and soil nitrate content related to nitrous oxide production and emission. In small, real-world observations, the KGML-ag turns out to be much more accurate than either ecosys or pure machine learning models and is 1,000 times faster than previously used computational models.
Compared to GHGs such as carbon dioxide and methane, nitrous oxide is not as well-known, but it is about 300 times more powerful than carbon dioxide in trapping heat in the atmosphere. Human-induced nitrous oxide emissions from agricultural synthetic fertilizer and cattle manure have also grown by at least 30 percent over the past four decades.
Researchers involved in the study were from the University of Minnesota, the University of Illinois at Urbana-Champaign, Lawrence Berkeley National Laboratory, and the University of Pittsburgh.
For more details, read the article in the University of Minnesota News and Events.
|
You might also like:
- GM Crops' Benefits to Fight Climate Change May Be Underestimated Than Previously Documented
- Genome Editing Offers Solutions to GHG Emission Problems
- Pocket K No. 43: Biotechnology and Climate Change
Biotech Updates is a weekly newsletter of ISAAA, a not-for-profit organization. It is distributed for free to over 22,000 subscribers worldwide to inform them about the key developments in biosciences, especially in biotechnology. Your support will help us in our mission to feed the world with knowledge. You can help by donating as little as $10.
-
See more articles:
-
News from Around the World
- Gene Discovery Transfers Rust Resistance to Wheat from Wild Relative
- Gene Drive Organisms: There is No One Size Fits All
- Machine Learning Model Could Help Reduce Agricultural Greenhouse Gas Emissions
- U.S. EPA and State of Florida Approve Oxitec's Second Pilot Project in the Florida Keys
- NASA Climate Scientist Cynthia Rosenzweig is the 2022 World Food Prize Laureate
- Experts Develop Plant Wearables to Measure Water Loss
- Philippines Prepares for Golden Rice Seed Deployment
- HB4® Wheat Gets Approval in Australia and New Zealand
- UK to Bring Forward a Genetic Technology Bill for Sustainable Agriculture
-
Research Highlights
- Cold Stress-responsive Gene from Ornamental Grass Provides Resilience to Environmental Stress
- Purslane as Green-bioreactor for Expression of Human Serum Albumin Gene
-
Plant
- Potato with Amylose-free Starch Developed thru CRISPR-Cas9 Editing
-
Read the latest: - Biotech Updates (July 9, 2025)
- Gene Editing Supplement (June 25, 2025)
- Gene Drive Supplement (February 22, 2023)
-
Subscribe to BU: - Share
- Tweet